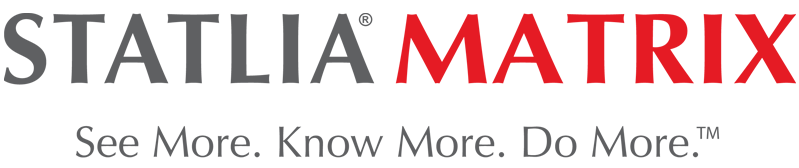

Weighting Assays
The weighting assays are the historical assays whose responses are used to compute the variance and weighting equations.
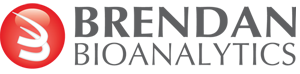
www.brendan.com
The weighting assays are the historical assays whose responses are used to compute the variance and weighting equations.
www.brendan.com